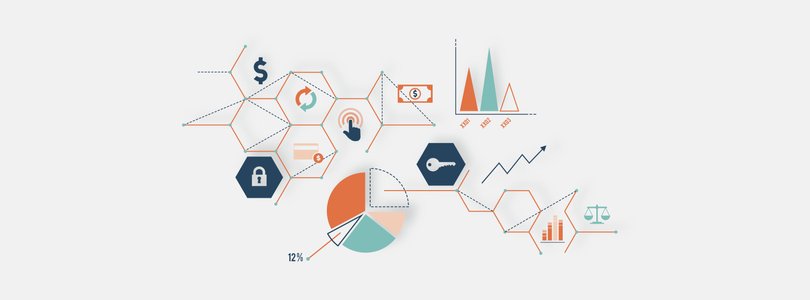
Reporting vs Analysis
11 Apr 2021 » Analytics Tips , Opinion
When people talk or think about Adobe Analytics, the first idea that comes to their minds tends to be “reporting”. In fact, this idea applies to any visualisation tool. However, this is only half of the story. Many forget that these tools actually have another, very important, capability: data analysis.
Reporting
They way I picture in my head the concept of reporting is structured data visualisation. Personally, I think that reporting is probably the most boring task you can do with Adobe Analytics. However, this is just my own view; I was chatting with a friend, who is working in a large corporation preparing dashboards, and she told me that she really liked it. I guess it is just a matter of preference. On the other hand, I have to admit that, presenting data in the best format can even be considered an art. The data has to convey a message and not everybody knows how to do it.
I have never been a fan of dashboards. In fact, when I worked as an Analytics consultant, I tried to avoid them as much as possible. This reminds me of an anecdote a client once told me. He was like me: he did not like dashboards. In his company, there was a manager who kept asking for the same reports regularly. He could not understand why this manager could not just log in to Adobe Analytics and pull the report himself. In the end, my client created a dashboard for this manager and never heard back from him.
What is undeniable is that reporting plays a big role in today’s data-driven organisations. Managers should take decisions based on data, not gut feeling.
Data Analysis
This is, to me, where the fun starts. If reporting is structured, data analysis is chaotic, the opposite. It is like treasure hunt: there is gold somewhere, but nobody knows where it is. You need to look for the cues in the data to get to some interesting results. You create multiple hypothesis, test them and, if you get nothing, discard them and start again.
Another important difference with reporting is that data analysis is open-ended. When building a dashboard, we have a clear idea of what we want. However, you never know where data analysis will take you. The results are likely to be something you had never thought of before.
I like to put an example that we once had at Adobe. We helped a client with an A/B test in Adobe Target. There were 2 experiences: the default and the contender. Once the test finished, the data showed that the default experience won. We could have stopped here and continued with other tests. However, upon further analysis, we saw that the contender did much better than the default experience for those visitors who were logged in. Since only a small percentage of visitors logged in, the data from the anonymous visitors had much more influence in the final results. Had we not analysed the data, this result would have been omitted. In the end, an XT activity was created, to show the new experience to logged-in visitors, leaving the default experience for anonymous visitors.
Finally, I would also add one additional activity to the data analysis basket: implementation debugging. I have done it a few times. Once you have finished the implementation, you realise that some data looks wrong. After looking at the typical suspects and finding nothing, you need to start looking elsewhere. Personally, I find very gratifying to analyse the data until I find the error. This process usually involves analysing the raw data with the typical Unix command-line tools: grep
, sed
, awk
…
I hope that, by now, it is clear the difference between the two aspects of Adobe Analytics and that both are equally important.
Image by rawpixel.com