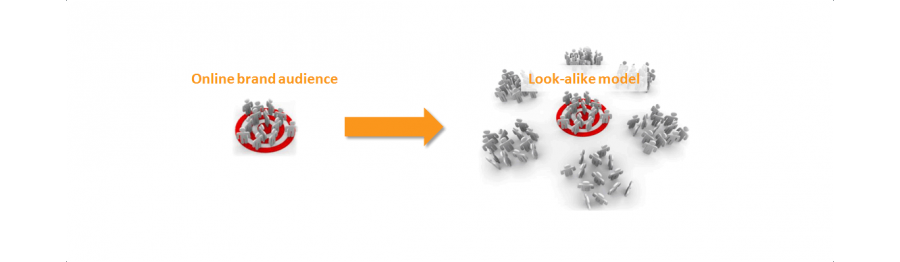
AAM, surveys and look alike modelling
08 Oct 2015 » AAM
As all digital marketers know, surveys provide invaluable information from visitors. They allow you to know various types of information from the visitors: the website itself, likelihood of buying, preferred products… The outcome of these surveys can be used to modify certain aspects of the experience or target the visitors with specific messages. All marketers would like every single customer to perform a survey and use that information to create a perfect experience for each visitor, but the reality is far from this ideal. Only very few visitors end up accepting the invitation and this usually happens when there is a potential reward.
Enter Adobe Audience Manager. One of its capabilities is the look alike modelling. Basically, this feature compares a base population with the rest of the population, finding similarities. You can think of it as an algorithm that gets all the traits from the base population, remove the base trait, and checks the rest of the population for visitors exhibiting the new list of traits. The main goal of this feature is to uncover hidden population segments and this is exactly what we need it for.
Going back to the survey, many on-line survey tools have the capability of processing the answers and provide a score or a classification. With this information, once the user finishes it (or after selecting a particular answer in a question), we can add a tracking pixel, with a key/value pair different for each score or classification. Creating a set of traits from this tracking pixel is trivial.
The next step is to create one model using one of these traits. I am not going to talk today how to use this functionality; that is material for another post. The algorithm will extract a subset of the population that looks very similar to the people who have conducted the survey, even if they have not conducted this survey. In fact, the algorithm generates a trait, that can be used in a segment.
After this explanation, let’s try to illustrate it in an example.
- Bank website
- 20,000,000 registered users
- A survey is created to analyse investment interest
- 5,000 people conduct the survey
- 1,000 people are classified as “interested in investing”
- A model is created to find similar people to those “interested in investing”
- The algorithm, with an accuracy of 0.6, finds 1,000,000 visitors potentially “interested in investing”
- These 1,000,000 visitors are then targeted with a campaign to show the investing products the bank has
In other words, from just a population of 1,000 visitors that we know for sure are interested in investing, we have uncovered a population 1,000 times bigger of potential investors, just by looking at similar traits.